Before going to war, each General has to research his opponents: how large their army is, what their weapons are, how many fights they have had, and what key tactics they use. This expertise will make it possible for the General to devise the correct plan and be ready for war.
Much like that, before going to big data, any decision-maker needs to know what they’re working with. Here, Platingnum addresses 7 global big data problems and proposes their solutions. Using this ‘inside knowledge,’ you’ll be able to take down frightening big data monsters without letting them defeat you in the fight to create a data-driven enterprise.
Obstacle #1: Insufficient Knowledge and Recognition of Big Data
Companies often do not really know the basics: what big data is, what its advantages are, what technology is required, etc. Without a simple understanding, a large data acquisition project risks being doomed to failure. Companies can spend a lot of time and money on items they don’t really know how to use.
And if workers do not appreciate the importance of big data and/or do not wish to alter internal procedures in order to implement it, they will resist it and hinder the company’s growth.
Solution:
Big data, a big transition for an organization, should be embraced first by top management and then down the ladder. In order to ensure the awareness and adoption of big data at all levels, IT departments need to arrange a number of training sessions and workshops.
In order to ensure ever greater adoption of big data, the application and use of the current big data solution must be supervised and managed. However, top managers should not overdo regulation because it could have a detrimental impact.
Obstacle #2: Cofounding the Variety of Big Data Technology
It can be possible to get lost in the multitude of big data applications that are now available on the market. Do you require Spark, or will the pace of Hadoop MapReduce be enough? Is it best to store the data in Cassandra or HBase? Finding the answers could be tricky. And it’s much easier to make a bad choice if you explore the ocean of technological opportunities without a good vision of what you need.
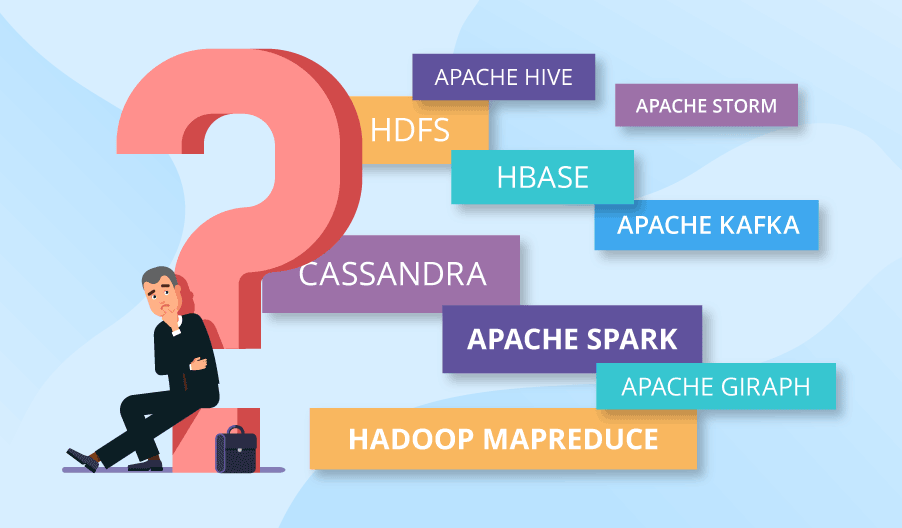
Solution:
If you’re new to the world of big data, wanting to get expert support will be the best way to go. You may recruit a specialist or switch to a Big Data Consultancy Provider, like us (Platingnum). In all scenarios, with collaborative efforts, you will be able to hammer out a plan and, on that basis, select the technology stack you need.
Obstacle #3: Paying Loads of Money
Projects for the implementation of big data include a lot of expenditures. If you go for an on-site solution, you’ll have to consider the costs of new hardware, new staff (administrators and developers), utilities, and so on. Plus: while the necessary frameworks are open-source, you will also have to pay for the development, setup, configuration and maintenance of new software.
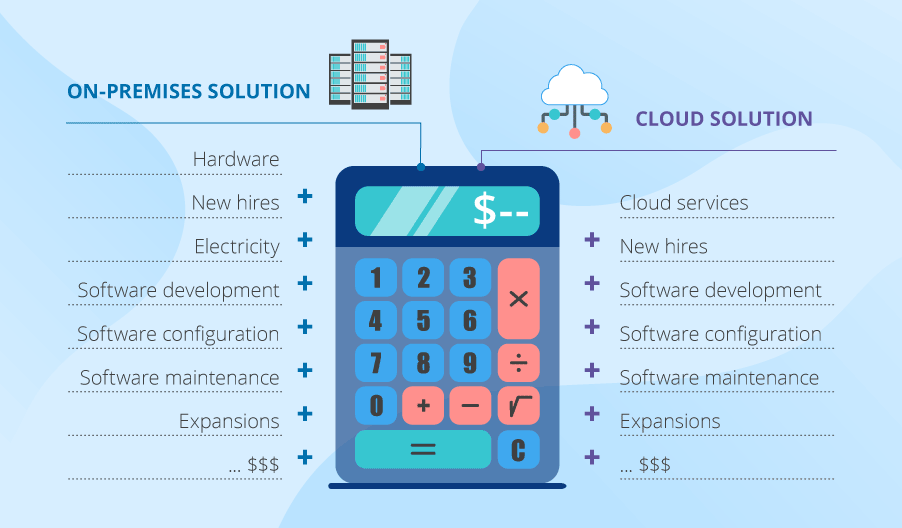
If you settle on a cloud-based Big Data approach, you will also need to recruit employees (as above) and pay for cloud services, the implementation of big data solutions, as well as the configuration and management of the necessary frameworks.
Moreover, in both cases, you’re supposed to have to really encourage potential expansions to stop big data development getting out of hand and costing you a lot.
Solution:
The precise redemption of your company’s pocket would rely on your company’s specific technological needs and market objectives. For example, businesses that want simplicity will benefit from the cloud. When businesses with incredibly tough security requirements will go on-site.
Hybrid solutions are also available where portions of the data are analysed in cloud and parts – on-premises, which may also be cost-effective. And using data lakes or algorithm optimizations (if performed correctly) can also save money:
- Data lakes: can provide inexpensive storage of data that you don’t need to analyse at the moment.
- Optimized algorithms: in particular, will reduce computational power consumption by 5 to 100 times. Or even more so.
All in all, the trick to overcoming this problem is to carefully analyse your needs and choose the appropriate course of action.
Obstacle #4: Managing Complexities of Data Quality
Data from a variety of sources
Sooner or later, you can run into the data integration challenge, when the data you need to interpret comes from a variety of sources in a variety of different formats. For example, eCommerce firms need to review data from website records, call centres, competitor website scans and social media. Obviously, the data types may be different, and their matching can be troublesome.
Unreliable data
Nobody is hiding the fact that big data isn’t 100% reliable. And it’s not that important, all in all. But that doesn’t mean you’re not supposed to monitor how accurate the data is. Not only does it contain incorrect facts, but it can also duplicate itself and contain inconsistencies. And it is doubtful that data of incredibly poor quality will offer any valuable perspectives or glossy prospects to your daunting market activities.
Solution:
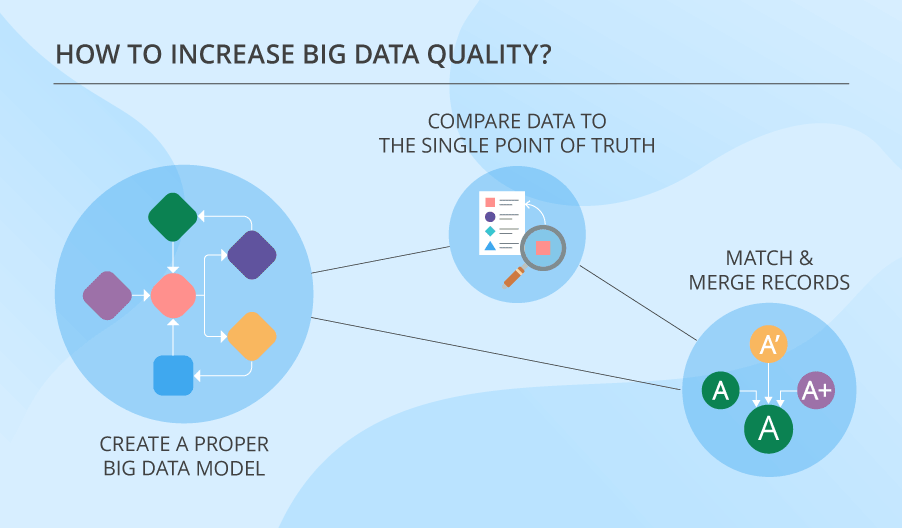
There’s a whole bunch of techniques used to clean up records. But first things, first. Your big data needs to have the right model. Only after you create that, you can go ahead and do other stuff, like:
- Compare details to a single truth point (for instance, compare variants of addresses to their spellings in the postal system database).
- Match and merge data, whether they belong to the same person.
But keep in mind that big data is never 100% reliable. You need to know it and deal with it, which is something that this article on big data quality will help you do.
Obstacle #5: Risky Big Data Security Holes
The security issues of big data are quite a big problem that requires a whole other article on the topic. So let’s dig at the issue on a bigger scale.
Big data acquisition projects also bring security back to later stages. And, honestly, this isn’t that much of a smart decision. Big data technologies are evolving, but their security capabilities are still neglected, although it is hoped that security can be provided at the application level. And what are we going to get? Both times (with technological development and project implementation) big data security is being thrown aside.
Solution:
Precautionary action against your potential big data security threats puts security first. It is especially relevant at the stage of developing the architecture of your solution. And if you don’t get along with big data security right from the start, it’s going to bite you when you least expect it.
Obstacle #6: Tricky Method of Turning Big Data to Useful Insights
Here’s an example: your super-cool big data analytics looks at what item pairs customers buy (say, needle and thread) based solely on your past consumer behavior data. Meanwhile, on Instagram, a soccer player puts on his latest look, and the two characteristic items he’s wearing are the white Nike sneakers and the beige cap. It looks amazing in them, and the people who see it tend to dress this way, too. They hurry to buy a matching pair of shoes and a similar cap. But you just have shoes in your store. As a consequence, you’re losing money, and maybe some regular clients.
Solution:
The explanation why you did not have the necessary goods in stock is that your big data tool does not analyse data from social networks or from rival online stores. And your rival’s big data, among other items, shows developments in social media in near-real time. And their store has both pieces, and also gives a 15% discount if you purchase them both.
The theory here is that you ought to build a proper system of variables and data sources, the study of which would provide the necessary insights to guarantee that nothing is out of range. Such a method should also contain external databases, although it can be difficult to collect and interpret external data.
Obstacle #5: Upscaling Problems
The most characteristic trait of big data is its dramatic capacity to evolve. And this is precisely one of the most serious problems of big data.
The architecture of your solution can be thought about and modified to upscaling without any additional effort. The real challenge, though, is not the actual method of adding new processing and storage capacities. It’s because of the difficulty of scaling up so that the system’s efficiency doesn’t decline and you remain under the budget.
Solution:
The first and foremost precaution for problems like these is the proper design of your big data approach. As long as you can boast about such a big data approach, less challenges are expected to emerge later. Another really important thing to do is plan your big data algorithms while having your future upscaling in mind.
But in addition, you will need to prepare for the maintenance and support of the infrastructure and ensure that all updates relating to data development are adequately addressed. And, on top of that, conducting systematic performance assessments will help recognise and correct weak points in a timely manner.
Win or Lose
As you might have observed, most of the problems reviewed can be anticipated and addressed if the big data approach has a decent, well-organized and thought-provoking architecture. This suggests that businesses should take a strategic approach to it. Although apart from that, the firms should:
- Hold seminars for workers to ensure the acceptance of large results.
- Carefully choose the technology stack.
- Mind expenses and a potential upscaling scheme.
- Remember that the data is not 100% reliable, but also manages its accuracy.
- Dig deep and big into actionable perspectives.
- Never ignore the security of big data.
If the business practises these tips, it has a good chance of defeating these top Seven.
Pingback: What is the Quality of Your Big Data? Dirty, Clean, or Cleanish! | Platingnum Big data
Pingback: Big Data: A Stairway to Heaven or A Highway to Hell? | Platingnum Big data