If you think that with big data, you’re going to cast a spell and easily improve your company, take off your cloak and throw away your wand, because big data isn’t magic. But if you roll up your sleeves and do some sweeping, it will do a trick and help you produce a spectacular business outcome.
Big data is strong and powerful, but not too perfect. This article reveals that it faces many problems and that data quality is one of them. Many companies understand these challenges and resort to big data services to deal with them. But why precisely do they do so, if big data is never 100% accurate? And how high is the output of big data? That you’re going to find out in this article.
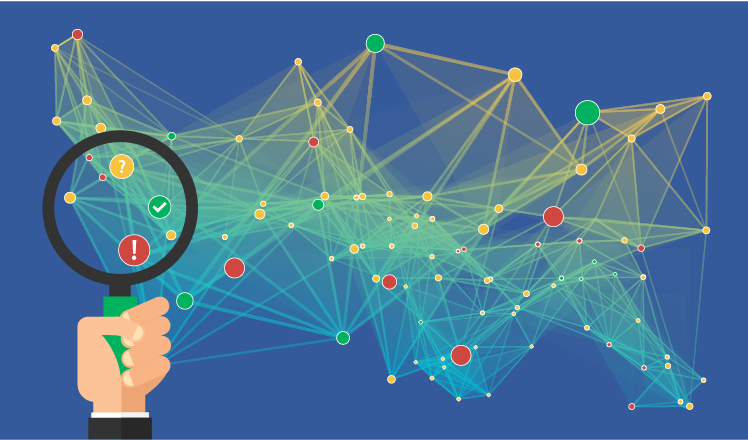
What if Your Big Data is of bad Quality?
Relatively poor quality of the big data can be either incredibly dangerous or not as serious. Here’s an indication of this. If your big data platform analyses the behaviour of the user on your website, you would, of course, want to know the real state of things. And you can do so. But maintaining 100 percent reliable tourist experience logs would not be sufficient just to see a big picture. It wouldn’t even be feasible, in reality.
However, if the Big Data Analytics tracks real-time data from, for example, let’s say, hospital heart monitors, 3% of the error margin could mean that you have not saved someone’s life.
So, everything here depends on the business in particular. And on a particular task, sometimes. And that means that before you rush to drive the data to the maximum possible degree of accuracy, you need to pause for a second. First, you can assess the big data quality requirements and then determine how high your big data quality needs to be.
What Exactly Meant by Good Quality?
We need a series of parameters to differentiate poor or dirty data from decent or clean data. It should be noted, though, that these refer to data quality as a whole, without connection with big data exclusively.
There are a variety of standards set when it comes to data quality, but Platingnum have chosen 5 of the most critical data characteristics to ensure that the data is safe.
- Consistency – the rational relations: There should be no anomalies in the correlated data sets, such as duplication, contradictions, and gaps. For example, it should be difficult to have two identical IDs for two separate workers or to refer to a non-existent entry in another row.
- Accuracy – the true state of affairs: The data should be accurate, continuous and focus on how things really are. All estimates based on this data display the true outcome.
- Completeness – all the elements needed: Your data is possibly made up of several components. In this situation, you need to include all the interdependent elements to ensure that the data will be interpreted correctly. For example, you have a lot of sensor data, but there is no information about the exact position of the sensor. You’re not even going to be able to explain how the factory equipment works and what affects this action.
- Auditability – maintenance and management: Data itself and the data management process as a whole should be structured in such a manner that you can conduct data quality audits on a daily or on-demand basis. This would help maintain a greater degree of data adequacy.
- Orderliness – configuration and format: Data should be ordered in a certain order. It needs to conform with all the data format specifications, structure, range of appropriate values, basic market rules and so on. For example, the temperature in the oven must be determined in Fahrenheit and cannot be-14 °F.
If you’re having trouble recalling the requirements, here’s a principle that may help: all of their first letters together make the word ‘cacao’.
Difference with Big Data Quality
If we talk specifically about big data, we have to note: not all of these conditions apply to big data and not all of them are 100%-achievable.
The problem with consistency is that the specific characteristics of big data make ‘noise’ possible in the first place. The vast scale and structure of big data make it impossible to erase any of it. It’s also needless sometimes. However, in some situations, logical relationships within the big data must be in place. For example, if a bank’s big data tool detects possible fraud. The Big Data platform will track your social networks. And you should confirm whether you’re on holiday in the UK. In other words, it relates information about you from various data sets and thus needs a certain degree of accuracy (an accurate link between your bank account and your social network accounts).
Whereas, at the same time as gathering feedback about a single commodity in social networks, duplication and inconsistencies would be appropriate. Some people can have several accounts and use them at various times, in the first case they claim they like the stuff, and in the second case they dislike it. Why is it all right? Since it won’t change the performance of the big data analytics on a wide scale.
As far as accuracy is concerned, we have already outlined in the article that its level differs from task to task. Picture a situation: you need to review the information from the previous month and the data for 2 days vanishes. You can’t really quantify any correct numbers without this evidence. Even if we’re talking about TV advertisers’ opinions, it’s not that critical: without them, we can still measure monthly averages and patterns. However, if the problem is more complex and more complex measurements or comprehensive statistical information are required (as in the case of a heart monitor), incorrect data will lead to incorrect decisions and even more errors.
Completeness isn’t so much to think about, too, because big data inevitably comes with a lot of holes. So it’s all right. In the same situation, when the 2-day data has vanished, we will still get good research results because of a large amount of other related data. Even without this measly component, the entire picture would still be sufficient.
Big data has resources for audibility. If you want to inspect the consistency of your big data, you may. However, the organisation will need time and money for this. For example, to build scripts that would verify the accuracy of the data and run these scripts, which can be expensive due to large data volumes.
And now to the orderliness. You should obviously be prepared for a degree of ‘controllable chaos’ in your records. For example, data lakes typically pay little attention to data structure and value adequacy. They just store what they’re getting. However, before data is placed into large data centres, it normally undergoes a cleaning process that will partly assure the accuracy of your data. But only in part.
Should You Stay ‘Dirty’ or go ‘Clean’?
As you can see, none of these big data quality standards are rigid or appropriate in all situations. And tailoring the big data approach to fulfil all of these to the utmost extent may:
- Cost a lot of it.
- It takes a lot of time.
- Scale down the efficiency of the system.
- Well, be quite impossible.
That’s why certain businesses are neither chasing clean data nor sticking to the dirty one. They’re going for ‘good enough data‘. This suggests that they set a minimum acceptable criterion that would give them sufficient analytical performance. And then they make sure that their data output is still above it.
How to Improve the Quality of Big Data?
We have three rules of thumb for you to consider when agreeing on your big data quality strategy and when running all other data quality control procedures:
Rule 1: Be careful about the source of the data. You should have a specific hierarchy of data source reliability and not all of them have similarly decent knowledge. Data from free or comparatively insecure sources should always be verified. A social network is a prime example of such a dubious source of data:
- It might not be easy to track the time that a single social media event has occurred.
- You can’t be sure of the source of the knowledge mentioned
- Or it could be difficult for algorithms to understand the feelings expressed in user posts.
Rule 2: Organize proper storage and transformation. Your data lakes and data warehouses need to be looked at if you want decent data quality. And a reasonably ‘solid’ data-cleaning mechanism has to be in operation when the data is being moved from a data lake to a large data warehouse. In addition, at this stage, the data has to be compared to all other information used to achieve a certain degree of accuracy (if needed at all).
Rule 3: Hold periodic audits. This one we’ve already discussed, so it merits some extra time. Software quality audits, as well as an audit of the big data solution, are an integral part of the maintenance process. You may need manual and automated audits. For example, you can evaluate your data quality issues and write scripts that run on a routine basis and inspect your data quality problem areas. If you have no expertise in such matters, or if you are unsure if you have all the tools you need, you may try outsourcing the data quality audits.
Pingback: Top 7 Big Data Challenges and How to Solve Them | Platingnum Big data